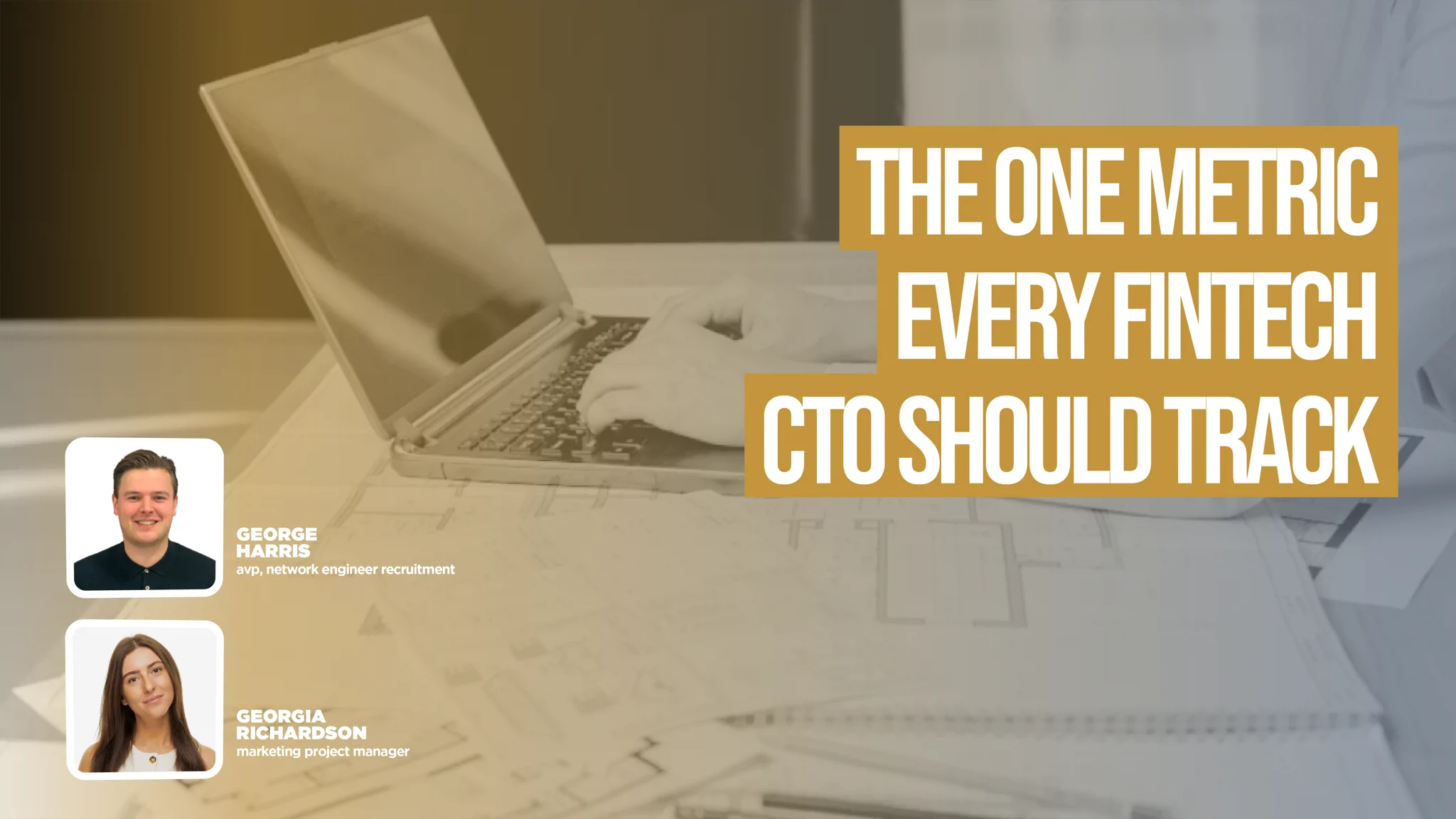
Download your free copy of the latest Financial Technologist magazine here.
There is a major disconnect between companies’ ambitions to leverage artificial intelligence (AI) and their preparedness. The requisite foundations have largely not been laid in order to confidently implement AI strategies - yielding new and faster insights. Success requires more than just sophisticated algorithms and computing power; it demands a keen focus on the fundamentals of data quality, the intricacies of data connectivity, and the principles of governance.
At ADI, we’ve heard from data, product, and technology executives across the Financial Services and Technology industries (among many more) that, despite size, implementation, or use case differences, they are still struggling with the fundamentals. This gap contributes to why CDOs have short shelf lives, data projects have varying degrees of success, and the organisations that have gotten the fundamentals right are significantly ahead of the pack.
Here are some of the best practices we’ve collected from executives and leaders who are driving successful AI and data initiatives at their organisations.
Elevate Data Quality
Data quality is the cornerstone of effective AI. Poor-quality data can mislead AI models, leading to inaccurate predictions and unreliable insights. To improve AI outcomes, organisations must establish rigorous data quality management practices. This includes implementing standardised data collection procedures, conducting thorough data cleaning, and ensuring data accuracy and consistency. Advanced techniques such as anomaly detection can automate the identification of data quality issues, facilitating timely corrections. By investing in data quality, organisations can build a solid foundation for AI models, resulting in more precise and trustworthy insights.
Augment Data Connectivity
The ability of AI to generate meaningful insights is significantly enhanced by understanding the relationships and connections between disparate data points. Entity resolution, the process of identifying and linking multiple records that refer to the same entity across different databases, is pivotal in achieving this. It enables a more comprehensive view of the data, allowing AI systems to make connections that were previously obscured by silos.
By integrating entity resolution techniques, organisations can enrich their datasets, providing AI models with the context needed to produce more nuanced and accurate analyses. This connectivity is crucial for applications ranging from customer relationship management to fraud detection, where understanding relationships between entities can uncover valuable insights.
Implement Robust Data Governance
Data governance encompasses the policies, standards, and procedures that ensure the effective and secure management of data assets. In the context of AI, robust data governance is essential not only for maintaining data quality but also for ensuring ethical AI practices. Effective governance frameworks address data privacy, security, and compliance with regulations such as GDPR and CCPA, thereby safeguarding sensitive information and maintaining public trust. Furthermore, governance practices should include mechanisms for metadata management and data lineage tracking, which provides transparency into the data sources, transformations, and uses within AI models. This transparency is crucial for validating AI outputs and facilitating accountability.
Embrace Data from Outside Your Organisation
Beyond improving individual data quality and resolving entities, integrating multiple data sources significantly amplifies AI's analytical capabilities. Inclusion of data from outside the immediate organisation allows AI models to draw on a broader spectrum of information, enhancing their ability to detect patterns, trends, and insights that would be invisible in more limited datasets. Effective data integration requires careful mapping of data schemas, resolving inconsistencies, and ensuring seamless data flow between systems. Techniques such as data virtualisation can provide real-time access to integrated datasets, further accelerating the speed to insight.
The path to enhancing AI results and performance is intricately linked to the quality, connectivity, and governance of data. By prioritising these aspects, organisations can ensure that their AI initiatives are built on a foundation of trust, reliability, and stability. As we advance into the future, the focus must remain on harnessing the power of data through core fundamentals, ensuring that AI or the next leading advancement fulfil their promise to drive positive transformation.