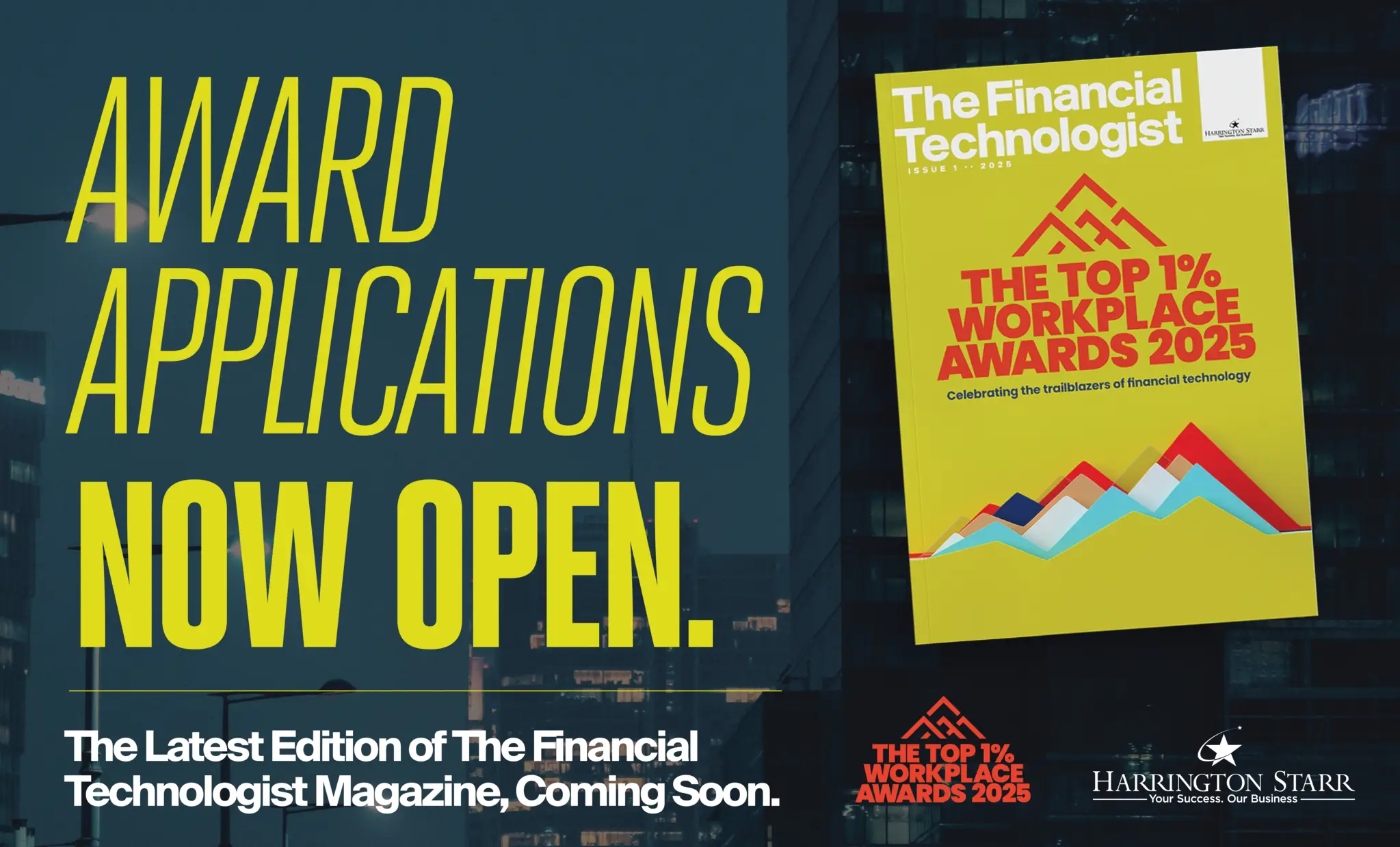
Download your free copy of the latest Financial Technologist magazine here.
Staying ahead of market trends and making sound financial decisions is no small feat. It requires analytical acumen, intuition, and deep industry expertise. Traditionally, financial analysts have relied on experience, manual research, and intuition to guide their analyses. However, the rapid advancement of artificial intelligence (AI) and machine learning is transforming this landscape, offering powerful tools to enhance and streamline workflows.
One groundbreaking concept driving this change is the Chain of Thought (CoT) approach. CoT enables AI models to break down complex tasks into logical, step-by-step processes, mimicking how human analysts tackle intricate problems. By adopting this structured workflow, AI systems can produce faster, more refined results while preserving the depth of analysis.
In this article, we’ll explore how AI and Chain of Thought reasoning are reshaping financial analysis. We’ll walk through a real-world case study and uncover actionable insights you can apply to your workflows.
What is Chain of Thought Reasoning?
Chain of Thought (CoT) is a reasoning technique where AI breaks down a complex task into smaller, logical steps. Think of it as the digital equivalent of how a seasoned analyst systematically works through a challenging problem.
For example, instead of jumping straight to conclusions, CoT-powered AI models first dissect the problem, analyse each component, and provide intermediate reasoning before arriving at a decision. This process ensures both speed and accuracy, which are critical in financial analysis.
Case Study: Building a Workflow to Analyse AI Adoption Trends
To illustrate the potential of AI and CoT workflows, let’s examine a real-world example. Our journey began with a simple question: How can we systematically understand the types of AI solutions companies are prioritising? To tackle this, we used a Large Language Model (LLM) to create a structured framework — a Chain of Thought workflow requiring minimal human input.
Here’s how it unfolded:
Step 1: Create a Mindmap and Summaries
We started by identifying a core theme: AI Solutions. Using an LLM, we generated a detailed mindmap.
For instance, the theme branched into categories like Machine Learning Applications, further breaking down into subtopics such as Predictive Maintenance, Fraud Detection, and Customer Segmentation.
For each node in the mindmap, the LLM also generated summaries — concise descriptions or questions contextualised by the taxonomy. For example, under Predictive Maintenance, the model produced 20 sentences explaining its relevance in the context of AI and machine learning applications.
Step 2: Perform Content Retrieval
With the mindmap and summaries in place, we conducted a multi-threaded similarity search using the Bigdata.com Enterprise retrieval system. This enabled us to retrieve high-quality content from targeted datasets like news articles, earnings call transcripts or company filings. For our use case, we specifically concentrated on earnings call transcripts.
By specifying time ranges, company watchlists, and sector focus, we mimicked how experienced analysts scan documents for insights—only faster and at scale.
Step 3: Content Labeling
To ensure accuracy, we implemented a verification layer. The AI assigned labels to content chunks based on the taxonomy, enabling both top-down (theme-to-detail) and bottom-up (detail-to-theme) analysis.
For example, a document mentioning Predictive Maintenance was cross-referenced with summaries to confirm its relevance. This step minimised AI hallucinations and improved precision.
Step 4: Results Visualisation
The analysis revealed fascinating insights. For instance:
- 19% of companies discussing AI solutions in earnings calls referenced chatbots
- 18% highlighted customer segmentation
- 17% mentioned data entry automation
Breaking it down by sector, we found:
- Energy and Utilities: Predictive Maintenance led the pack
- Telecommunications: Chatbots dominated discussions
- Financials: Chatbots, Data Entry Automation, and Fraud Detection emerged as top applications
Step 5: Assess Financial Materiality
Finally, we connected these insights to financial materiality at the company level. Using the LLM, we identified key financial KPIs for each AI initiative and ranked companies based on alignment with measurable financial outcomes.
For example, consider American Airlines. During an earnings call, their executives highlighted predictive maintenance initiatives. Below, we provide a verbatim quote from the call to demonstrate how these statements tie back to measurable metrics.
Using the Bigdata API, we extracted sentences linked to the identified KPIs, enabling hard numbers extractions to support financial analysts in evaluating company valuations.
In this case, we uncovered valuable insights related to maintenance cost per flight hour and utilisation rates of predictive maintenance tools and technologies — key metrics for assessing the financial impact of these initiatives.
Why It Matters
The integration of AI and Chain of Thought workflows is reshaping the role of financial analysts in profound ways. Mainly, these tools:
- Automate routine tasks like document scanning and data retrieval.
- Reduce errors through structured reasoning and robust verification.
- Enable analysts to focus on high-value activities, like interpreting trends and making strategic recommendations.
The bigger picture: the possibilities extend far beyond AI solutions. By adjusting the initial seed input, this workflow can be applied to other themes such as sustainability, emerging technologies, or sector-specific trends.
Key Takeaways for Financial Analysts
- Adopt AI Early: early adopters gain a competitive edge in an increasingly data-driven market
- Focus on Financial Impact: use AI insights to link industry trends directly to financial KPIs
- Tailor Workflows to Your Needs: AI workflows like CoT can be customised to suit various analytical challenges
What’s Next?
These are transformative times for financial analysts. By embracing AI-driven workflows, you can unlock smarter decisions, greater precision, and a significant competitive advantage.
By Peter Hafez, Chief Data Scientist, RavenPack
Download your free copy of the latest Financial Technologist magazine here.